copied
Readme
Files and versions
5.3 KiB
Image-Text Retrieval Embdding with CLIP
author: David Wang
Description
This operator extracts features for image or text with CLIP which can generate embeddings for text and image by jointly training an image encoder and text encoder to maximize the cosine similarity.
Code Example
Load an image from path './teddy.jpg' to generate an image embedding.
Read the text 'A teddybear on a skateboard in Times Square.' to generate an text embedding.
Write the pipeline in simplified style:
import towhee
towhee.glob('./teddy.jpg') \
.image_decode() \
.image_text_embedding.clip(model_name='clip_vit_base_patch16', modality='image') \
.show()
towhee.dc(["A teddybear on a skateboard in Times Square."]) \
.image_text_embedding.clip(model_name='clip_vit_base_patch16', modality='text') \
.show()


Write a same pipeline with explicit inputs/outputs name specifications:
import towhee
towhee.glob['path']('./teddy.jpg') \
.image_decode['path', 'img']() \
.image_text_embedding.clip['img', 'vec'](model_name='clip_vit_base_patch16', modality='image') \
.select['img', 'vec']() \
.show()
towhee.dc['text'](["A teddybear on a skateboard in Times Square."]) \
.image_text_embedding.clip['text','vec'](model_name='clip_vit_base_patch16', modality='text') \
.select['text', 'vec']() \
.show()
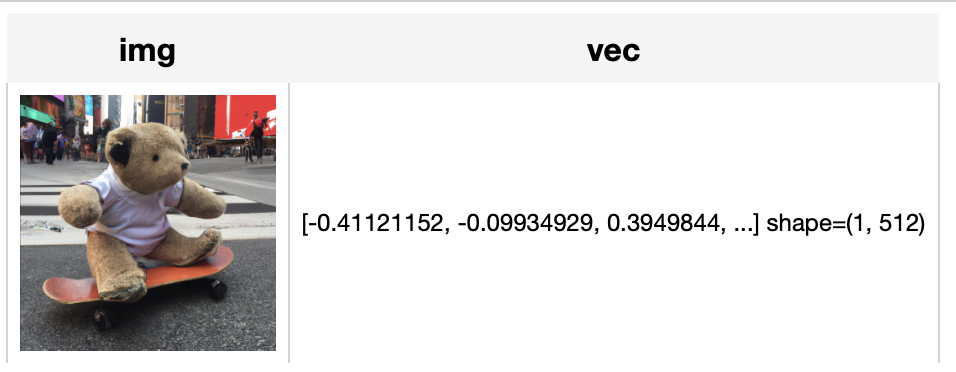

Factory Constructor
Create the operator via the following factory method
clip(model_name, modality)
Parameters:
model_name: str
The model name of CLIP. Supported model names:
- clip_vit_base_patch16
- clip_vit_base_patch32
- clip_vit_large_patch14
- clip_vit_large_patch14_336
modality: str
Which modality(image or text) is used to generate the embedding.
checkpoint_path: str
The path to local checkpoint, defaults to None.
If None, the operator will download and load pretrained model by model_name
from Huggingface transformers.
Interface
An image-text embedding operator takes a towhee image or string as input and generate an embedding in ndarray.
save_model(format='pytorch', path='default')
Save model to local with specified format.
Parameters:
format: str
The format of saved model, defaults to 'pytorch'.
path: str
The path where model is saved to. By default, it will save model to the operator directory.
from towhee import ops
op = ops.image_text_embedding.clip(model_name='clip_vit_base_patch16', modality='image').get_op()
op.save_model('onnx', 'test.onnx')
Parameters:
data: towhee.types.Image (a sub-class of numpy.ndarray) or str
The data (image or text based on specified modality) to generate embedding.
Returns: numpy.ndarray
The data embedding extracted by model.
supported_model_names(format=None)
Get a list of all supported model names or supported model names for specified model format.
Parameters:
format: str
The model format such as 'pytorch', 'torchscript'.
from towhee import ops
op = towhee.ops.image_text_embedding.clip(model_name='clip_vit_base_patch16', modality='image').get_op()
full_list = op.supported_model_names()
onnx_list = op.supported_model_names(format='onnx')
print(f'Onnx-support/Total Models: {len(onnx_list)}/{len(full_list)}')
Fine-tune
Requirement
If you want to train this operator, besides dependency in requirements.txt, you need install these dependencies.
! python -m pip install datasets evaluate
Get start
import towhee
clip_op = towhee.ops.image_text_embedding.clip(model_name='clip_vit_base_patch16', modality='image').get_op()
data_args = {
'dataset_name': 'ydshieh/coco_dataset_script',
'dataset_config_name': '2017',
'cache_dir': './cache',
'max_seq_length': 77,
'data_dir': path_to_your_coco_dataset,
'image_mean': [0.48145466, 0.4578275, 0.40821073],
"image_std": [0.26862954, 0.26130258, 0.27577711]
}
training_args = {
'num_train_epochs': 3, # you can add epoch number to get a better metric.
'per_device_train_batch_size': 8,
'per_device_eval_batch_size': 8,
'do_train': True,
'do_eval': True,
'remove_unused_columns': False,
'output_dir': './tmp/test-clip',
'overwrite_output_dir': True,
}
Dive deep and customize your training
You can change the training script in your customer way. Or your can refer to the original hugging face transformers training examples.
5.3 KiB
Image-Text Retrieval Embdding with CLIP
author: David Wang
Description
This operator extracts features for image or text with CLIP which can generate embeddings for text and image by jointly training an image encoder and text encoder to maximize the cosine similarity.
Code Example
Load an image from path './teddy.jpg' to generate an image embedding.
Read the text 'A teddybear on a skateboard in Times Square.' to generate an text embedding.
Write the pipeline in simplified style:
import towhee
towhee.glob('./teddy.jpg') \
.image_decode() \
.image_text_embedding.clip(model_name='clip_vit_base_patch16', modality='image') \
.show()
towhee.dc(["A teddybear on a skateboard in Times Square."]) \
.image_text_embedding.clip(model_name='clip_vit_base_patch16', modality='text') \
.show()


Write a same pipeline with explicit inputs/outputs name specifications:
import towhee
towhee.glob['path']('./teddy.jpg') \
.image_decode['path', 'img']() \
.image_text_embedding.clip['img', 'vec'](model_name='clip_vit_base_patch16', modality='image') \
.select['img', 'vec']() \
.show()
towhee.dc['text'](["A teddybear on a skateboard in Times Square."]) \
.image_text_embedding.clip['text','vec'](model_name='clip_vit_base_patch16', modality='text') \
.select['text', 'vec']() \
.show()
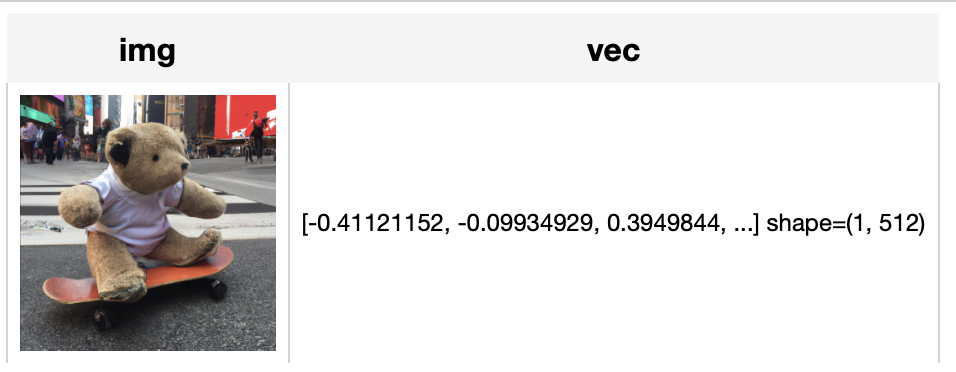

Factory Constructor
Create the operator via the following factory method
clip(model_name, modality)
Parameters:
model_name: str
The model name of CLIP. Supported model names:
- clip_vit_base_patch16
- clip_vit_base_patch32
- clip_vit_large_patch14
- clip_vit_large_patch14_336
modality: str
Which modality(image or text) is used to generate the embedding.
checkpoint_path: str
The path to local checkpoint, defaults to None.
If None, the operator will download and load pretrained model by model_name
from Huggingface transformers.
Interface
An image-text embedding operator takes a towhee image or string as input and generate an embedding in ndarray.
save_model(format='pytorch', path='default')
Save model to local with specified format.
Parameters:
format: str
The format of saved model, defaults to 'pytorch'.
path: str
The path where model is saved to. By default, it will save model to the operator directory.
from towhee import ops
op = ops.image_text_embedding.clip(model_name='clip_vit_base_patch16', modality='image').get_op()
op.save_model('onnx', 'test.onnx')
Parameters:
data: towhee.types.Image (a sub-class of numpy.ndarray) or str
The data (image or text based on specified modality) to generate embedding.
Returns: numpy.ndarray
The data embedding extracted by model.
supported_model_names(format=None)
Get a list of all supported model names or supported model names for specified model format.
Parameters:
format: str
The model format such as 'pytorch', 'torchscript'.
from towhee import ops
op = towhee.ops.image_text_embedding.clip(model_name='clip_vit_base_patch16', modality='image').get_op()
full_list = op.supported_model_names()
onnx_list = op.supported_model_names(format='onnx')
print(f'Onnx-support/Total Models: {len(onnx_list)}/{len(full_list)}')
Fine-tune
Requirement
If you want to train this operator, besides dependency in requirements.txt, you need install these dependencies.
! python -m pip install datasets evaluate
Get start
import towhee
clip_op = towhee.ops.image_text_embedding.clip(model_name='clip_vit_base_patch16', modality='image').get_op()
data_args = {
'dataset_name': 'ydshieh/coco_dataset_script',
'dataset_config_name': '2017',
'cache_dir': './cache',
'max_seq_length': 77,
'data_dir': path_to_your_coco_dataset,
'image_mean': [0.48145466, 0.4578275, 0.40821073],
"image_std": [0.26862954, 0.26130258, 0.27577711]
}
training_args = {
'num_train_epochs': 3, # you can add epoch number to get a better metric.
'per_device_train_batch_size': 8,
'per_device_eval_batch_size': 8,
'do_train': True,
'do_eval': True,
'remove_unused_columns': False,
'output_dir': './tmp/test-clip',
'overwrite_output_dir': True,
}
Dive deep and customize your training
You can change the training script in your customer way. Or your can refer to the original hugging face transformers training examples.