copied
Readme
Files and versions
Updated 10 months ago
image-captioning
Image Captioning with CapDec
author: David Wang
Description
This operator generates the caption with CapDec which describes the content of the given image. ExpansionNet v2 introduces the Block Static Expansion which distributes and processes the input over a heterogeneous and arbitrarily big collection of sequences characterized by a different length compared to the input one. This is an adaptation from DavidHuji/CapDec.
Code Example
Load an image from path './image.jpg' to generate the caption.
Write a pipeline with explicit inputs/outputs name specifications:
from towhee.dc2 import pipe, ops, DataCollection
p = (
pipe.input('url')
.map('url', 'img', ops.image_decode.cv2_rgb())
.map('img', 'text', ops.image_captioning.capdec(model_name='capdec_noise_0')
.output('img', 'text')
)
DataCollection(p('./image.jpg')).show()
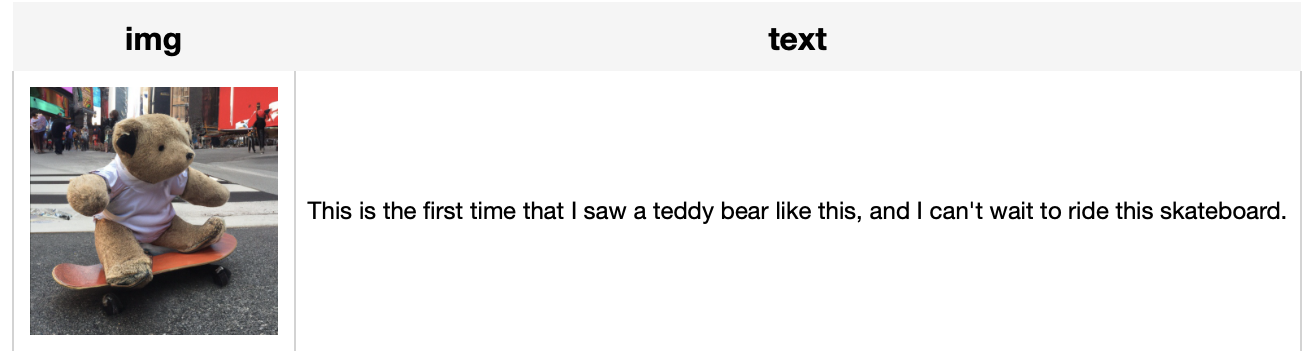
Factory Constructor
Create the operator via the following factory method
capdec(model_name)
Parameters:
model_name: str
The model name of CapDec. Supported model names:
- capdec_noise_0
- capdec_noise_01
- capdec_noise_001
- capdec_noise_0001
Interface
An image captioning operator takes a towhee image as input and generate the correspoing caption.
Parameters:
data: towhee.types.Image (a sub-class of numpy.ndarray)
The image to generate caption.
Returns: str
The caption generated by model.
More Resources
- What is a Generative Adversarial Network? An Easy Guide: Just like we classify animal fossils into domains, kingdoms, and phyla, we classify AI networks, too. At the highest level, we classify AI networks as "discriminative" and "generative." A generative neural network is an AI that creates something new. This differs from a discriminative network, which classifies something that already exists into particular buckets. Kind of like we're doing right now, by bucketing generative adversarial networks (GANs) into appropriate classifications. So, if you were in a situation where you wanted to use textual tags to create a new visual image, like with Midjourney, you'd use a generative network. However, if you had a giant pile of data that you needed to classify and tag, you'd use a discriminative model.
- Multimodal RAG locally with CLIP and Llama3 - Zilliz blog: A tutorial walks you through how to build a multimodal RAG with CLIP, Llama3, and Milvus.
- Supercharged Semantic Similarity Search in Production - Zilliz blog: Building a Blazing Fast, Highly Scalable Text-to-Image Search with CLIP embeddings and Milvus, the most advanced open-source vector database.
- The guide to clip-vit-base-patch32 | OpenAI: clip-vit-base-patch32: a CLIP multimodal model variant by OpenAI for image and text embedding.
- The guide to gte-base-en-v1.5 | Alibaba: gte-base-en-v1.5: specialized for English text; Built upon the transformer++ encoder backbone (BERT + RoPE + GLU)
- Multimodal RAG with Milvus and GPT-4o: Join us for a webinar for a demo of multimodal RAG with Milvus and GPT-4o
- An LLM Powered Text to Image Prompt Generation with Milvus - Zilliz blog: An interesting LLM project powered by the Milvus vector database for generating more efficient text-to-image prompts.
- From Text to Image: Fundamentals of CLIP - Zilliz blog: Search algorithms rely on semantic similarity to retrieve the most relevant results. With the CLIP model, the semantics of texts and images can be connected in a high-dimensional vector space. Read this simple introduction to see how CLIP can help you build a powerful text-to-image service.
| 5 Commits | ||
---|---|---|---|
|
1.1 KiB
|
3 years ago | |
|
4.2 KiB
|
10 months ago | |
|
679 B
|
3 years ago | |
|
14 KiB
|
2 years ago | |
|
4.1 KiB
|
2 years ago | |
|
16 KiB
|
3 years ago | |
|
0 B
|
3 years ago | |
|
183 KiB
|
2 years ago |