copied
Readme
Files and versions
Updated 9 months ago
image-captioning
Image Captioning with ExpansionNet v2
author: David Wang
Description
This operator generates the caption with ExpansionNet v2 which describes the content of the given image. ExpansionNet v2 introduces the Block Static Expansion which distributes and processes the input over a heterogeneous and arbitrarily big collection of sequences characterized by a different length compared to the input one. This is an adaptation from jchenghu/ExpansionNet_v2.
Code Example
Load an image from path './image.jpg' to generate the caption.
Write a pipeline with explicit inputs/outputs name specifications:
from towhee import pipe, ops, DataCollection
p = (
pipe.input('url')
.map('url', 'img', ops.image_decode.cv2_rgb())
.map('img', 'text', ops.image_captioning.expansionnet_v2(model_name='expansionnet_rf'))
.output('img', 'text')
)
DataCollection(p('./image.jpg')).show()
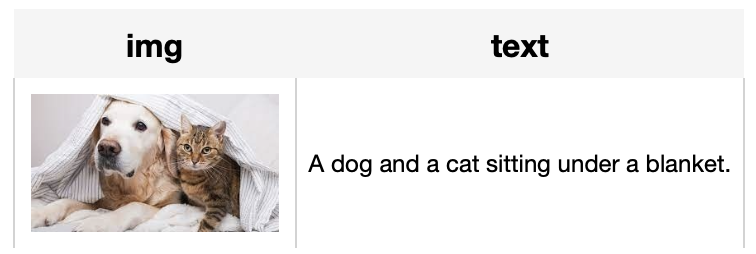
Factory Constructor
Create the operator via the following factory method
expansionnet_v2(model_name)
Parameters:
model_name: str
The model name of ExpansionNet v2. Supported model names:
- expansionnet_rf
Interface
An image captioning operator takes a towhee image as input and generate the correspoing caption.
Parameters:
data: towhee.types.Image (a sub-class of numpy.ndarray)
The image to generate caption.
Returns: str
The caption generated by model.
More Resources
- What is a Transformer Model? An Engineer's Guide: A transformer model is a neural network architecture. It's proficient in converting a particular type of input into a distinct output. Its core strength lies in its ability to handle inputs and outputs of different sequence length. It does this through encoding the input into a matrix with predefined dimensions and then combining that with another attention matrix to decode. This transformation unfolds through a sequence of collaborative layers, which deconstruct words into their corresponding numerical representations. At its heart, a transformer model is a bridge between disparate linguistic structures, employing sophisticated neural network configurations to decode and manipulate human language input. An example of a transformer model is GPT-3, which ingests human language and generates text output.
- The guide to instructor-xl | HKU NLP: instructor-xl: an instruction-finetuned model tailored for text embeddings with the best performance when compared to
instructor-base
andinstructor-large.
- The guide to voyage-large-2 | Voyage AI: voyage-large-2: general-purpose text embedding model; optimized for retrieval quality; ideal for tasks like summarization, clustering, and classification.
- The guide to instructor-large | HKU NLP: instructor-large: an instruction-finetuned model tailored for text embeddings; better performance than
instructor-base
, but worse thaninstructor-xl
. - OpenAI text-embedding-3-large | Zilliz: Building GenAI applications with text-embedding-3-large model and Zilliz Cloud / Milvus
- The guide to clip-vit-base-patch32 | OpenAI: clip-vit-base-patch32: a CLIP multimodal model variant by OpenAI for image and text embedding.
- Understanding ImageNet: A Key Resource for Computer Vision and AI Research: The large-scale image database with over 14 million annotated images. Learn how this dataset supports advancements in computer vision.
- What is a Generative Adversarial Network? An Easy Guide: Just like we classify animal fossils into domains, kingdoms, and phyla, we classify AI networks, too. At the highest level, we classify AI networks as "discriminative" and "generative." A generative neural network is an AI that creates something new. This differs from a discriminative network, which classifies something that already exists into particular buckets. Kind of like we're doing right now, by bucketing generative adversarial networks (GANs) into appropriate classifications. So, if you were in a situation where you wanted to use textual tags to create a new visual image, like with Midjourney, you'd use a generative network. However, if you had a giant pile of data that you needed to classify and tag, you'd use a discriminative model.
- Zilliz partnership with PyTorch - View image search solution tutorial: Zilliz partnership with PyTorch
| 14 Commits | ||
---|---|---|---|
|
3 years ago | ||
|
3 years ago | ||
|
3 years ago | ||
|
1.1 KiB
|
3 years ago | |
|
4.9 KiB
|
9 months ago | |
|
733 B
|
2 years ago | |
|
10 KiB
|
3 years ago | |
|
238 KiB
|
3 years ago | |
|
6.1 KiB
|
2 years ago | |
|
44 B
|
3 years ago | |
|
90 KiB
|
3 years ago |